The AI Revolution: A Game-Changer for Business and Society
Artificial Intelligence (AI) is reshaping our world in profound ways. From streamlining business processes to enhancing decision-making capabilities, AI is changing how we work, live, and interact with technology.
The potential impact of AI on the global economy is staggering.
PwC estimates that AI could contribute up to $15.7 trillion to the global economy by 2030. This figure represents countless innovations, improved efficiencies, and new opportunities for growth across virtually every sector.
As we embrace these technologies, we must also grapple with the ethical implications and potential challenges they present. Job displacement concerns, data privacy issues, and the need for responsible AI development are just a few of the complex questions we need to address as a society.
In this comprehensive exploration, we’ll dive into the fascinating world of AI-automation, data assist, and services. We’ll uncover both the immense potential and the critical considerations that come with this technological revolution.
Articles similar to this one can be found at: https://aiismsforbeginners.com/
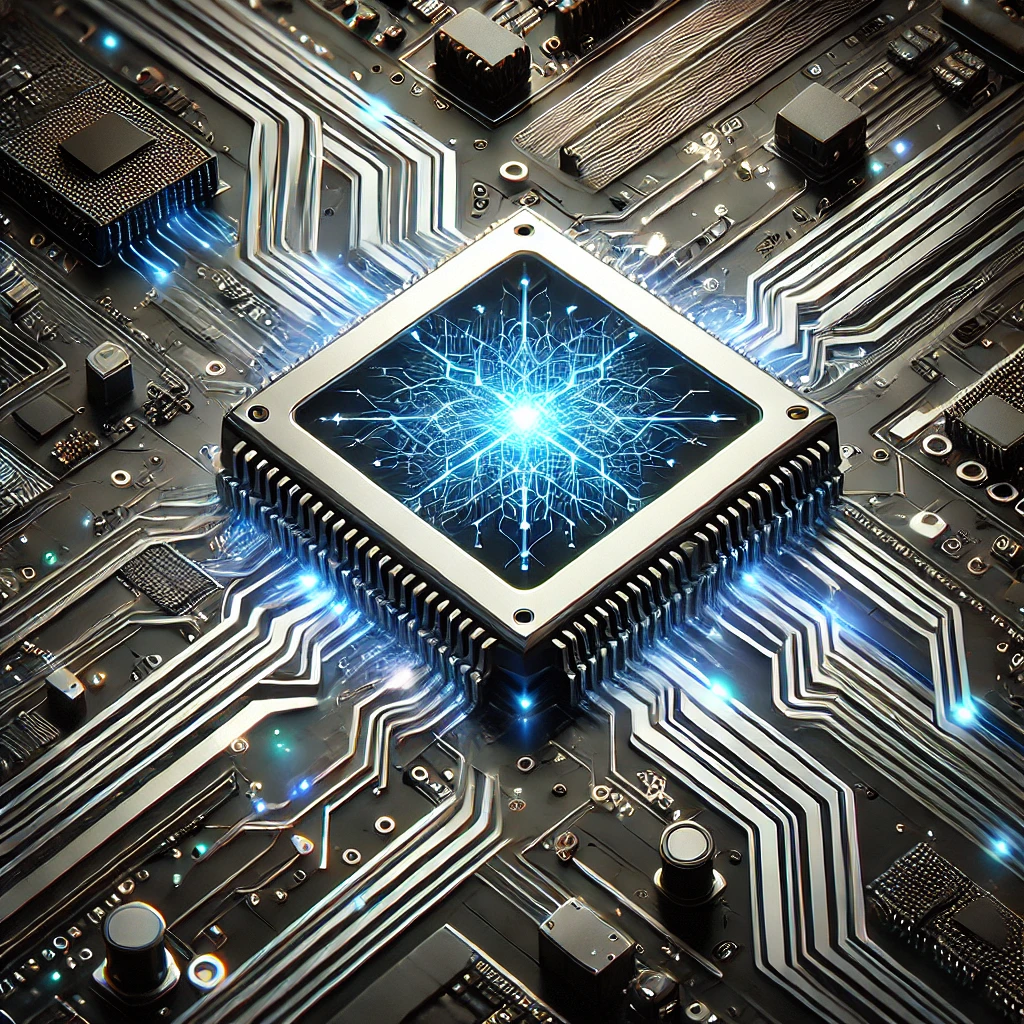
Intelligent Automation: Revolutionizing Industries
Intelligent automation stands at the forefront of the AI revolution. By combining artificial intelligence with automation, we’ve created systems that can learn, adapt, and make decisions.
This technology is transforming industries by taking over repetitive tasks, analyzing large amounts of data, and even making complex decisions.
Do you want to make some AI-assisted income? Check this out: https://afflat3e3.com/trk/lnk/2095F12B-EF3C-40ED-A493-33D18EAF413E/?o=29080&c=918277&a=747225&k=87E61794FE43FFD1E4754AC553099D77&l=32738
Manufacturing: A New Era of Efficiency
In manufacturing, AI-powered robots are now capable of performing repetitive tasks and adapting to changes in their environment. Computer vision systems can identify defects in products with greater accuracy than human inspectors, leading to improved quality control and reduced waste.
For example, BMW has implemented AI-powered quality control systems in its production lines. These systems use machine learning algorithms and high-resolution cameras to detect even the tiniest imperfections in car bodies and paint jobs.
This level of precision has significantly reduced defects and improved overall product quality.
A great AI-assisted money-making program to begin with is here: https://afflat3e3.com/trk/lnk/2095F12B-EF3C-40ED-A493-33D18EAF413E/?o=29080&c=918277&a=747225&k=87E61794FE43FFD1E4754AC553099D77&l=32738
Financial Services: Enhanced Security and Risk Assessment
In the financial sector, AI is changing fraud detection and risk assessment. Machine learning algorithms can analyze patterns in transaction data to identify potentially fraudulent activities in real-time, protecting both businesses and consumers.
These systems are constantly learning and improving, staying one step ahead of sophisticated fraudsters.
JPMorgan Chase, for instance, has developed an AI system called COiN (Contract Intelligence) that can review commercial loan agreements in seconds, a task that previously took lawyers and loan officers 360,000 hours annually. This saves time and reduces errors and improves consistency in contract analysis.
Choose from many different AI-assisted programs to earn money effortlessly: https://afflat3e3.com/trk/lnk/2095F12B-EF3C-40ED-A493-33D18EAF413E/?o=29080&c=918277&a=747225&k=87E61794FE43FFD1E4754AC553099D77&l=32738
The Job Displacement Conundrum
The rise of intelligent automation raises valid concerns about job displacement. While it’s true that some jobs may become obsolete, history has shown that technological advancements often create new job opportunities.
The key comes from reskilling and upskilling the workforce to adapt to these changes.
A report by the World Economic Forum forecasts that while 75 million jobs may be displaced by AI and automation by 2022, 133 million new jobs will be created. These new roles will likely focus on tasks that need human creativity, emotional intelligence, and complex problem-solving skills – areas where AI still lags behind human capabilities.
Choose from many different AI-assisted programs to earn money effortlessly: https://afflat3e3.com/trk/lnk/2095F12B-EF3C-40ED-A493-33D18EAF413E/?o=29080&c=918277&a=747225&k=87E61794FE43FFD1E4754AC553099D77&l=32738
Data Assistance: Turning Information into Actionable Intelligence
AI-powered data assistance is transforming raw data into actionable intelligence across various sectors. This capability is proving invaluable in fields ranging from healthcare to business strategy.
Healthcare: AI as a Powerful Diagnostic Tool
One of the most exciting applications of data assistance is in healthcare. AI algorithms can analyze medical images, patient records, and research papers to assist in diagnosis, treatment planning, and drug discovery.
A study published in Nature demonstrated that AI can forecast breast cancer up to five years in advance, outperforming traditional models. The AI system, developed by researchers at MIT, analyzed mammograms and patient histories to identify subtle patterns that human radiologists might miss.
This early detection capability could significantly improve patient outcomes and reduce healthcare costs.
Choose from many different AI-assisted programs to earn money effortlessly: https://afflat3e3.com/trk/lnk/2095F12B-EF3C-40ED-A493-33D18EAF413E/?o=29080&c=918277&a=747225&k=87E61794FE43FFD1E4754AC553099D77&l=32738
Business Strategy: Predictive Analytics for Informed Decision-Making
In the business world, predictive analytics can forecast market trends, customer behavior, and potential risks. This allows businesses to stay ahead of the curve and make informed strategic decisions.
For example, Amazon uses AI-powered predictive analytics to optimize its supply chain and inventory management. The company’s algorithms analyze historical sales data, search queries, and even weather forecasts to predict demand for specific products in different regions.
This allows Amazon to stock its warehouses efficiently, reducing costs and improving delivery times.
Choose from many different AI-assisted programs to earn money effortlessly: https://afflat3e3.com/trk/lnk/2095F12B-EF3C-40ED-A493-33D18EAF413E/?o=29080&c=918277&a=747225&k=87E61794FE43FFD1E4754AC553099D77&l=32738
The Data Privacy Imperative
The power of data assistance comes with significant responsibilities. Ensuring data privacy and security is paramount, especially when dealing with sensitive information.
Companies must apply robust security measures and adhere to data protection regulations to maintain trust and protect people’ rights.
The European Union’s General Data Protection Regulation (GDPR) and similar laws worldwide have set new standards for data privacy. Companies using AI and data analytics must now be transparent about their data collection and usage practices, get explicit consent from users, and provide options for data deletion and portability.
AI tools are absolutely necessary to speed your learning. Try this resource to assist you: https://galaxy.ai/?ref=gary
AI-Powered Services: Enhancing User Experiences
From virtual assistants to personalized recommendations, AI-powered services are enhancing user experiences across various platforms.
Natural Language Processing: The Rise of Intelligent Assistants
Natural Language Processing (NLP) has enabled the development of sophisticated chatbots and virtual assistants that can understand and respond to human language with remarkable accuracy. These AI-powered tools are transforming customer service, providing instant support and freeing up human agents to handle more complex queries.
For instance, Bank of America’s virtual assistant, Erica, uses NLP to help customers with various banking tasks. Erica can understand natural language queries, provide account information, offer financial advice, and even help with bill payments.
Since its launch, Erica has assisted millions of customers, handling routine inquiries and freeing up human staff for more complex issues.
This program allows you to start small, then advertise more to make more profits: https://afflat3e3.com/trk/lnk/2095F12B-EF3C-40ED-A493-33D18EAF413E/?o=29080&c=918277&a=747225&k=87E61794FE43FFD1E4754AC553099D77&l=32738
Entertainment: Personalized Content Recommendations
In the entertainment industry, recommendation systems powered by AI are changing how we find out about and consume content. Streaming platforms like Netflix and Spotify use machine learning algorithms to analyze user preferences and viewing habits, offering personalized recommendations that keep users engaged.
Netflix’s recommendation system is particularly sophisticated. It doesn’t just look at what you’ve watched, and when you watched it, how long you watched, and even at what point you might have stopped watching a show. This granular level of data analysis allows Netflix to offer highly personalized content suggestions, improving user satisfaction and retention.
The Challenge of Algorithmic Bias
The increasing reliance on AI-powered services raises questions about privacy and the potential for these systems to reinforce biases. It’s crucial to develop transparent AI systems that can explain their decision-making processes and to apply safeguards against algorithmic bias.
For example, in 2018, Amazon discovered that its AI-powered hiring tool was biased against women. The system had been trained on resumes submitted to the company over a 10-year period, most of which came from men.
As a result, the AI learned to prefer male candidates.
This case highlights the importance of carefully designing and monitoring AI systems to prevent the perpetuation of societal biases.
Implementing AI: A Step-by-Step Approach
Implementing AI in your organization can seem daunting, but with a structured approach, it’s achievable for businesses of all sizes. Here’s a detailed guide to get you started:
1. Identify the Problem
Start by clearly defining the business problem you want to solve with AI. This could be improving customer service, optimizing supply chain operations, or enhancing product recommendations.
Be specific about what you want to achieve and how you’ll measure success.
For example, a retail company might identify that they want to reduce customer churn by 20% over the next year using AI-powered personalized marketing campaigns.
2. Assess Data Availability
AI systems need large amounts of high-quality data to function effectively. Evaluate your current data sources and identify any gaps that need to be filled. Consider the following questions:
- What data do you now have?
- Is the data clean and well-organized?
- Do you have enough historical data to train AI models?
- Are there any privacy or legal concerns with using this data?
If you find gaps in your data, develop a plan to collect the necessary information. This might involve setting up new data collection processes or purchasing data from third-party providers.
3. Choose the Right AI Solution
Based on your problem and data availability, choose the suitable AI technology. This could range from machine learning algorithms to natural language processing tools.
Consider factors such as:
- The complexity of the problem
- The type of data you’re working with (structured vs. unstructured)
- The level of accuracy required
- The interpretability needs of the solution
For instance, if you’re working on a image recognition problem, you might choose a convolutional neural network (CNN). For a text classification task, you might opt for a recurrent neural network (RNN) or a transformer model like BERT.
4. Start Small
Begin with a pilot project to test the AI solution in a controlled environment. This allows you to assess its effectiveness and identify any issues before scaling up.
A pilot project should:
- Have a clear, limited scope
- Use a subset of your data
- Have defined success metrics
- Be time-bound (e.g., 3-6 months)
For example, if you’re implementing an AI-powered customer service chatbot, you might start by deploying it to handle a specific type of inquiry for a small segment of your customers.
5. Invest in Talent
Building an AI-powered system needs specialized skills. Consider hiring AI experts or partnering with AI service providers to fill any skill gaps in your team.
Key roles to consider include:
- Data Scientists
- Machine Learning Engineers
- AI Ethicists
- Domain Experts (who understand your business context)
You might also consider upskilling your existing team through training programs or partnerships with educational institutions.
6. Ensure Ethical Implementation
Develop guidelines for the ethical use of AI in your organization, addressing issues such as data privacy, transparency, and fairness. This might involve:
- Creating an AI ethics board
- Developing an AI ethics policy
- Implementing processes for regular ethical audits of AI systems
- Training all employees on AI ethics
Ethical AI isn’t about compliance – this involves building trust with your customers and stakeholders.
7. Monitor and Iterate
Continuously watch the performance of your AI system and be prepared to make adjustments based on feedback and changing business needs. Set up processes for:
- Regular performance reviews
- User feedback collection
- Model retraining and updating
- Monitoring for drift in data distributions or model performance
AI implementation is not a one-time project, but an ongoing process of learning and improvement.
AI tools are absolutely necessary to speed your learning. Try this resource to assist you: https://galaxy.ai/?ref=gary
Overcoming Common Problems in AI Implementation
While the potential of AI is immense, there are several common pitfalls, problems, issues, problems, issues, problems, issues that organizations should be aware of:
Overestimating AI Capabilities
AI is powerful, but it’s not magic. Be realistic about what AI can achieve and avoid setting unrealistic expectations.
AI excels at tasks involving pattern recognition and large-scale data analysis, but it struggles with tasks requiring common sense reasoning or emotional intelligence.
For example, while an AI system might be excellent at diagnosing certain medical conditions from images, it can’t replace the holistic approach of a human doctor who can consider a patient’s overall health, lifestyle, and emotional state.
Start off selling AI-assisted digital products here: https://afflat3e3.com/trk/lnk/2095F12B-EF3C-40ED-A493-33D18EAF413E/?o=29080&c=918277&a=747225&k=87E61794FE43FFD1E4754AC553099D77&l=32738
Neglecting Data Quality
AI systems are only as good as the data they’re trained on. Ensure your data is accurate, comprehensive, and representative to avoid biased or inaccurate results.
This involves:
- Regular data cleaning and preprocessing
- Checking for and addressing biases in your data
- Ensuring your data is representative of the real-world scenarios your AI will encounter
Remember the adage: “Garbage in, garbage out.” No matter how sophisticated your AI model is, it can’t overcome fundamentally flawed or biased data.
Ignoring Explainability
Some AI models, particularly deep learning systems, can be “black boxes” that are difficult to interpret. Prioritize explainable AI techniques to maintain transparency and trust.
This is particularly important in regulated industries or when AI is making decisions that significantly impact people’s lives.
For instance, in financial services, you might need to explain why an AI system denied a loan application. Using techniques like LIME (Local Interpretable Model-agnostic Explanations) or SHAP (SHapley Additive exPlanations) can help make complex AI models more interpretable.
Failing to Consider Ethical Implications
AI systems can perpetuate or even amplify existing biases if not carefully designed. Regularly assess your AI systems for fairness and potential negative impacts. This involves:
- Testing your AI on diverse datasets
- Monitoring for unintended consequences
- Considering the broader societal impacts of your AI system
For example, if you’re developing an AI system for hiring, confirm it’s not discriminating against certain groups based on protected characteristics like race, gender, or age.
Neglecting Human Expertise
While AI can automate many tasks, human expertise is still crucial. Strive for a balance between AI capabilities and human insight.
The most effective AI implementations often involve human-AI collaboration, where AI augments human capabilities as opposed to replacing them entirely.
For instance, in customer service, AI chatbots can handle routine inquiries, but complex issues or emotionally charged situations are still best handled by human agents.
Adapting AI to Different Scenarios
One of the most powerful aspects of AI is its versatility. Here are some detailed tips for adapting AI techniques to different scenarios:
Customize for Your Industry
Different industries have unique challenges and data types. Tailor your AI approach to your specific sector, whether it’s healthcare, finance, retail, or manufacturing.
In healthcare, for example, AI might be used for image analysis in radiology, predictive analytics for patient outcomes, or natural language processing for analyzing medical records. Each of these applications needs different AI techniques and considerations.
In retail, AI might be used for demand forecasting, personalized recommendations, or inventory management. The data types and business goals in retail are quite different from healthcare, necessitating a different approach to AI implementation.
Here is a great program for you to get started with:
Scale Appropriately
Not every problem needs a complex deep learning system. Sometimes, simpler machine learning techniques can be just as effective and more effective.
Consider the following factors when choosing your AI approach:
- The complexity of the problem
- The amount of data available
- The computational resources at your disposal
- The need for real-time processing
For instance, for a simple classification task with a small dataset, a decision tree or random forest algorithm might be more suitable (and easier to interpret) than a deep neural network.
Combine AI Techniques
Don’t limit yourself to a single AI approach. Combining techniques like machine learning, natural language processing, and computer vision can lead to more robust solutions.
For example, in autonomous vehicles, computer vision is used to interpret the visual environment, while machine learning algorithms make decisions about steering, acceleration, and braking. Natural language processing might be used for the vehicle’s voice control system.
Consider Edge Computing
For applications requiring real-time processing or dealing with sensitive data, edge AI (running AI models on local devices as opposed to in the cloud) can be a game-changer. Edge AI offers several advantages:
- Reduced latency for real-time applications
- Improved privacy as data doesn’t need to leave the device
- Ability to function without a network connection
For instance, smartphone-based face recognition systems often use edge AI to process images locally, ensuring user privacy and enabling quick response times.
Embrace Transfer Learning
This technique allows you to apply knowledge from one AI model to a different but related task, saving time and resources in training new models. Transfer learning is particularly useful when you have limited data for your specific task but can leverage models trained on larger, related datasets.
For example, in computer vision, you might start with a model pre-trained on a large dataset like ImageNet, and then fine-tune it for your specific image classification task. This approach can significantly reduce training time and improve performance, especially when working with limited data.
AI tools are absolutely necessary to speed your learning. Try this resource to assist you: https://galaxy.ai/?ref=gary
Building on Basics: From Novice to AI Master
Mastering AI is an ongoing process that builds on basic concepts. As you progress from basic understanding to advanced applications, you’ll find that each step opens up new possibilities:
Start with the Basics
Understanding basic concepts is crucial. Begin by learning about:
- Machine learning algorithms (supervised, unsupervised, reinforcement learning)
- Neural networks and deep learning
- Data preprocessing techniques
- Model evaluation metrics
Resources like Andrew Ng’s Machine Learning course on Coursera or the “Deep Learning” book by Ian Goodfellow, Yoshua Bengio, and Aaron Courville can provide a solid foundation.
Dive into Specialized Areas
Once you have a grasp of the basics, explore specific AI domains based on your interests and needs:
- Computer Vision: Learn about convolutional neural networks (CNNs) and image processing techniques.
- Natural Language Processing: Study techniques like word embeddings, recurrent neural networks (RNNs), and transformer models.
- Reinforcement Learning: Understand concepts like Q-learning and policy gradients.
Each of these areas has its own set of algorithms, best practices, and challenges. Choose the area that aligns best with your goals and dive deep.
Stay Updated
The field of AI is rapidly evolving. Regularly read research papers, attend conferences, and join in online courses to stay current.
Some ways to stay updated include:
- Following AI researchers and organizations on social media
- Subscribing to AI-focused newsletters
- Participating in AI competitions on platforms like Kaggle
- Attending local AI meetups or conferences
What’s cutting-edge today might be standard practice tomorrow. Continuous learning is essential in this fast-paced field.
Practice with Real-World Projects
Apply your knowledge to actual problems. Start with Kaggle competitions or contribute to open-source AI projects.
This hands-on experience is invaluable for deepening your understanding and building a portfolio.
For example, you might:
- Participate in a Kaggle competition to predict house prices
- Contribute to an open-source natural language processing library
- Build a personal project, like a recommendation system for your favorite type of media
Collaborate and Share Knowledge
Join AI communities, join in forums, and share your experiences. Learning from others and contributing to the community is crucial for growth.
Some ways to engage with the AI community include:
- Participating in AI-focused subreddits or Stack Overflow
- Attending AI hackathons
- Writing blog posts about your AI projects or insights
- Mentoring others who are just starting their AI journey
Teaching others is often the best way to solidify your own understanding.
Exercises to Reinforce Your AI Knowledge
To truly master AI concepts, practice is essential. Here are some detailed exercises to help reinforce your learning:
Data Preprocessing Challenge
Take a messy dataset and clean it up, handling missing values, outliers, and inconsistencies. This exercise will help you understand the importance of data quality and the challenges of working with real-world data.
Steps:
- Find a dataset with various data quality issues (e.g., on Kaggle or UCI Machine Learning Repository)
- Identify and document all data quality issues
- Develop a strategy for addressing each issue
- Implement your strategy using Python libraries like pandas
- Compare the cleaned dataset with the original, noting the improvements
Model Comparison
Implement many machine learning models on the same dataset and compare their performance. This exercise will help you understand the strengths and weaknesses of different algorithms.
Steps:
- Choose a dataset suitable for classification or regression
- Split the data into training and test sets
- Implement at least three different models (e.g., logistic regression, random forest, neural network)
- Train each model on the training data
- Evaluate each model on the test data using suitable metrics
- Analyze the results, considering factors like accuracy, training time, and interpretability
NLP Project
Build a simple chatbot or sentiment analysis tool using natural language processing techniques. This exercise will introduce you to the challenges and techniques specific to working with text data.
Steps:
- Choose a specific task (e.g., building a customer service chatbot or analyzing sentiment in product reviews)
- Collect or find an suitable dataset
- Preprocess the text data (tokenization, removing stop words, etc.)
- Implement your chosen NLP technique (e.g., bag-of-words, word embeddings)
- Train and evaluate your model
- Test your model with new, unseen text inputs
Computer Vision Task
Develop an image classification model using a popular dataset like MNIST or CIFAR-10. This exercise will help you understand the basics of working with image data and implementing convolutional neural networks.
Steps:
- Load the chosen dataset
- Preprocess the images (normalization, augmentation)
- Design and apply a convolutional neural network
- Train the model, monitoring for overfitting
- Evaluate the model on a test set
- Experiment with different architectures or hyperparameters to improve performance
Ethical AI Analysis
Evaluate an existing AI system for potential biases and suggest methods to mitigate them. This exercise will help you develop critical thinking skills about the ethical implications of AI.
Steps:
- Choose an existing AI system (e.g., a facial recognition system or a credit scoring algorithm)
- Research how the system works and what data it uses
- Identify potential sources of bias in the system
- Analyze the potential impacts of these biases
- Propose methods to detect and mitigate these biases
- Consider the broader ethical implications of the system’s use
People Also Asked
What is AI automation?
AI automation refers to the use of artificial intelligence technologies to automate tasks and processes that traditionally required human intervention. It combines machine learning, natural language processing, and other AI techniques to create systems that can learn, adapt, and make decisions autonomously.
How is AI used in data analysis?
AI is used in data analysis to process large volumes of data quickly, identify patterns and trends, make predictions, and generate insights. Machine learning algorithms can automatically detect anomalies, classify data, and even suggest actions based on the analysis.
What are some examples of AI-powered services?
AI-powered services include virtual assistants like Siri or Alexa, recommendation systems on platforms like Netflix or Amazon, chatbots for customer service, fraud detection systems in banking, and personalized marketing campaigns.
How does AI improve business efficiency?
AI improves business efficiency by automating repetitive tasks, providing real-time insights for decision-making, optimizing processes through predictive analytics, and enhancing customer experiences through personalization.
What are the ethical concerns surrounding AI?
Ethical concerns surrounding AI include privacy issues, potential job displacement, algorithmic bias, lack of transparency in AI decision-making, and the long-term societal impacts of widespread AI adoption.
How can businesses start implementing AI?
Businesses can start implementing AI by identifying specific problems AI could solve, assessing their data readiness, starting with small pilot projects, investing in AI talent or partnerships, and ensuring ethical guidelines are in place.
What skills are needed to work in AI?
Skills needed to work in AI include programming (especially Python), statistics and mathematics, machine learning algorithms, data preprocessing, model evaluation, and domain-specific knowledge. Soft skills like problem-solving and communication are also crucial.
How is AI changing the job market?
AI is changing the job market by automating certain tasks, creating new job roles (like AI ethicists or machine learning engineers), and increasing demand for skills in data analysis, programming, and AI development.
What is the difference between AI and machine learning?
AI is a broader concept of machines being able to carry out tasks in a way that we would consider “smart”. Machine learning is a subset of AI that focuses on the ability of machines to receive data and learn for themselves, changing algorithms as they learn more about the information they’re processing.
How secure is AI technology?
The security of AI technology varies depending on its implementation. While AI can enhance cybersecurity through advanced threat detection, AI systems themselves can be vulnerable to attacks like data poisoning or model inversion.
Ensuring AI security needs ongoing vigilance and the implementation of robust security measures.
Key Takeaways
- AI-automation, data assist, and services are transforming industries, offering unprecedented efficiency and insights.
- Implementing AI needs a structured approach, starting with problem identification and ending with continuous monitoring and iteration.
- Ethical considerations, including data privacy and algorithmic bias, are crucial when developing and deploying AI systems.
- Adapting AI to different scenarios involves customization, scaling, and combining various AI techniques.
- Mastering AI is an ongoing process that needs continuous learning, practice, and engagement with the AI community.
This post contains links. If you click on these links and make a purchase, I may earn a commission at no additional cost to you. Rest assured, I only recommend products or services I believe will add value to my readers.
Articles similar to this one can be found at: https://aiismsforbeginners.com/