Artificial intelligence (AI) has become an integral part of our daily lives, influencing everything from the recommendations we receive on streaming platforms to the voice assistants on our smartphones. As AI continues to improve and shape our world, it’s crucial to understand the key concepts, terminologies, and implications of this transformative technology.
Articles similar to this one can be found at: https://aiismsforbeginners.com/
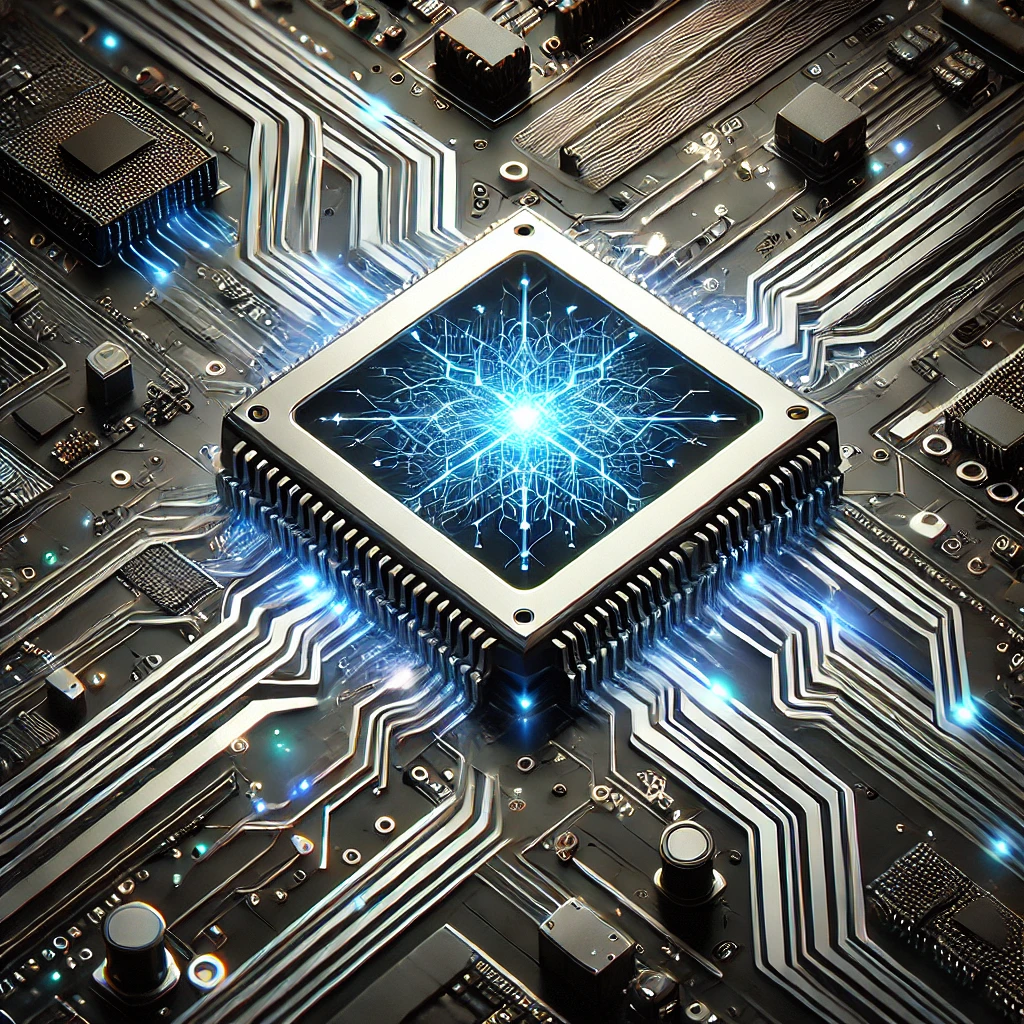
How many isms can you think of?
Capitalism Consumerism Baptism Socialism Fascism Totalitarianism Ultranationalism Communism Materialism Autism Stoicism Euphorism Populism Criticism Maoism Buddhism Mysticism Hinduism Marxism Racism Sexism Radicalism Terrorism Militarism Antisemitism
My point is this: There are quite a few “isms” in our world already. We are more familiar with some than we are with others. So with that in mind, is it not difficult to realize we have been and are currently in the growing awareness of AI-ism?
The Foundation of AI
Artificial intelligence refers to the development of computer systems capable of performing tasks that typically need human intelligence. These tasks include visual perception, speech recognition, decision-making, and language translation.
The field of AI encompasses several key technologies and approaches that form its foundation.
This subset of AI focuses on creating algorithms that can learn from data and make predictions or decisions without being explicitly programmed. Machine learning enables systems to improve their performance over time as they are exposed to more data.
There are three primary types of machine learning:
- Supervised Learning: This approach involves training an algorithm on a labeled dataset.
The algorithm learns to map input data to known output labels, much like a student learning under the guidance of a teacher.
For example, a supervised learning algorithm might be trained on a dataset of images labeled as “cat” or “dog” to learn how to classify new, unseen images.
- Unsupervised Learning: In this method, the algorithm works with unlabeled data, attempting to find patterns or structures within it.
Clustering algorithms, which group similar data points together, are a common example of unsupervised learning.
This approach can reveal hidden patterns in data that humans might not have noticed.
- Reinforcement Learning: This type of machine learning involves an agent learning to make decisions by interacting with an environment.
The agent receives rewards or penalties based on its actions, allowing it to learn optimal behavior over time.
Reinforcement learning has been successfully applied in areas such as game playing and robotics.
Neural Networks and Deep Learning
Neural networks are a class of machine learning algorithms inspired by the structure and function of the human brain. These networks consist of interconnected nodes (neurons) organized in layers. Each connection between neurons has a weight that decides its importance in the network’s decision-making process.
Deep learning is a subset of machine learning that uses neural networks with many layers, hence the term “deep.” These deep neural networks can automatically learn hierarchical representations of data, making them particularly effective for tasks like image and speech recognition. Deep learning has led to significant breakthroughs in AI, enabling systems to achieve human-level performance in various complex tasks.
Natural Language Processing (NLP)
Natural Language Processing focuses on enabling computers to understand, interpret, and generate human language. NLP technologies power chatbots, language translation services, and voice assistants.
Recent advancements in NLP, such as transformer models like GPT (Generative Pre-trained Transformer), have dramatically improved machines’ ability to process and generate human-like text.
Computer Vision
Computer vision deals with how computers gain high-level understanding from digital images or videos. This field combines elements of machine learning, optics, and image processing to enable machines to interpret and analyze visual information.
Computer vision technologies are used in facial recognition systems, autonomous vehicles, and medical image analysis, among many other applications.
A great cost-saving tool you need can be found at: https://galaxy.ai/?ref=gary
AI in Action: Real-World Applications
The theoretical concepts of AI come to life in many practical applications across various industries. Let’s explore some of the most impactful areas where AI is making a difference:
Healthcare Transformation
AI is improving healthcare through improved diagnostics, personalized treatment plans, and drug discovery. AI algorithms can analyze medical images to detect diseases like cancer at early stages, often with accuracy rivaling or surpassing human experts.
For example, deep learning models have been developed that can identify skin cancer from photographs with a level of accuracy comparable to dermatologists.
AI is also accelerating drug discovery by predicting how different chemical compounds will interact with biological targets. This can significantly reduce the time and cost associated with developing new medications.
Additionally, AI-powered systems are being used to analyze electronic health records and identify patterns that can lead to more personalized and effective treatments for patients.
Financial Intelligence
In the financial sector, AI powers algorithmic trading, fraud detection, and personalized banking experiences. Machine learning models can analyze large amounts of financial data to identify market trends and make investment decisions in milliseconds.
This high-frequency trading has become a significant part of global financial markets.
AI is also enhancing fraud detection capabilities in banking and finance. By analyzing patterns in transaction data, AI systems can identify potentially fraudulent activities in real-time, helping to protect consumers and financial institutions.
Furthermore, AI-powered chatbots and virtual assistants are providing personalized financial advice and customer service, improving the overall banking experience for consumers.
Education Transformation
AI is personalizing education through adaptive learning platforms. These systems can tailor content and pacing to individual students’ needs, potentially improving learning outcomes and efficiency.
For example, AI-powered tutoring systems can identify areas where a student is struggling and provide targeted exercises and explanations to help them improve.
AI is also being used to automate administrative tasks in education, such as grading many-choice tests or detecting plagiarism in written assignments. This frees up educators’ time, allowing them to focus more on direct interaction with students and higher-level teaching activities.
A great cost-saving tool you need can be found at: https://galaxy.ai/?ref=gary
Creative AI
AI is making significant strides in creative fields, challenging our understanding of creativity and art. AI systems can now generate original artwork, compose music, and even write poetry.
For example, the AI art generator DALL-E can create unique images based on text descriptions, while AI music composition tools can generate original melodies and harmonies.
This development raises fascinating questions about the nature of creativity and the role of human artists in an AI-augmented world. While some worry that AI might replace human creativity, others see it as a tool that can enhance and inspire human creative expression.
Ethical Considerations and Challenges
As AI becomes more powerful and pervasive, it brings with it a host of ethical considerations and challenges that society must grapple with:
AI Bias and Fairness
One of the most pressing issues in AI development is the potential for bias. AI systems can inadvertently perpetuate or even amplify existing societal biases if they’re trained on biased data or designed without consideration for diverse perspectives.
For example, facial recognition systems have been found to perform less accurately for certain racial groups, leading to concerns about fairness and discrimination.
Researchers are developing techniques for detecting and reducing bias in AI systems. This includes methods for auditing training data, developing more diverse datasets, and creating algorithms that can identify and correct for potential biases.
Ensuring fairness and equity in AI systems is crucial for their responsible deployment in society.
Privacy Concerns
As AI systems become more sophisticated in analyzing personal data, concerns about privacy and data protection are growing. The ability of AI to process large amounts of information and draw insights from it raises questions about data ownership, consent, and the potential for misuse.
For instance, AI-powered surveillance systems can track individuals’ movements and behaviors, potentially infringing on personal privacy. Similarly, AI algorithms used in social media can analyze users’ data to make highly accurate predictions about their preferences and behaviors, raising concerns about data exploitation.
Addressing these privacy concerns needs a combination of technological solutions, such as privacy-preserving machine learning techniques, and regulatory frameworks that protect individuals’ data rights.
AI Safety and Control
As AI systems become more autonomous and powerful, ensuring their safety and maintaining human control becomes crucial. This includes developing robust testing methodologies, fail-safe mechanisms, and clear protocols for AI deployment in critical systems.
The concept of AI alignment – ensuring that AI systems’ goals and behaviors align with human values and intentions – is a key area of research in AI safety. This involves technical challenges and philosophical and ethical questions about how to define and apply human values in AI systems.
The AI Job Displacement Debate
There’s ongoing debate about AI’s impact on employment. While AI may automate certain jobs, it’s also expected to create new roles and enhance human productivity in others.
Some studies suggest that AI could automate up to 30% of work activities globally by 2030, potentially displacing millions of workers.
However, history shows that technological advancements often create new job categories and industries. The key comes from adapting our education systems and workforce training to prepare for an AI-augmented future.
This may involve a greater emphasis on skills that are complementary to AI, such as creativity, emotional intelligence, and complex problem-solving.
Emerging Frontiers in AI
As we look to the future, several exciting frontiers in AI research and development are emerging:
Explainable AI (XAI)
As AI systems become more complex, there’s a growing need for transparency in their decision-making processes. Explainable AI aims to create models that can provide clear, understandable explanations for their outputs, which is crucial for building trust and accountability.
XAI is particularly important in high-stakes domains like healthcare and finance, where understanding the reasoning behind AI decisions can be critical. Techniques in XAI include developing interpretable machine learning models, generating human-readable explanations for AI decisions, and creating visualization tools that help users understand AI reasoning.
Artificial General Intelligence (AGI)
While current AI systems excel at specific tasks, the holy grail of AI research is creating systems with human-like general intelligence. AGI would be able to understand, learn, and apply knowledge across a wide range of domains, potentially improving every aspect of our lives.
Developing AGI presents enormous technical challenges and raises profound philosophical and ethical questions. Some researchers believe AGI could be achieved within decades, while others think it may be centuries away or even impossible.
The potential impact of AGI on society is so significant that it’s crucial to consider its implications and potential risks well in advance.
AI and Quantum Computing
The intersection of AI and quantum computing holds immense potential. Quantum computers could potentially solve certain types of problems exponentially faster than classical computers, which could lead to breakthroughs in AI capabilities.
Quantum machine learning algorithms could potentially process complex datasets much faster than classical algorithms, enabling new applications in fields like drug discovery and materials science. However, practical quantum computers capable of outperforming classical computers for these tasks are still in development.
AI for Scientific Discovery
AI is increasingly being used to speed up scientific research and discovery. From predicting protein structures to discovering new materials, AI is helping scientists tackle complex problems and explore new frontiers of knowledge.
For example, DeepMind’s AlphaFold has made significant breakthroughs in predicting protein structures, a task that’s crucial for understanding diseases and developing new drugs. AI is also being used in climate science to improve weather predictions and model climate change scenarios.
You need the tools to integrate with the workings of Artificial Intelligence.
The tools you need can be found here: https://galaxy.ai/?ref=gary
Navigating the AI Landscape: Tips for Staying Informed
As AI continues to improve rapidly, staying informed can be challenging. Here are some strategies to help you navigate the AI landscape:
- Follow reputable AI news sources and academic publications.
Websites like MIT Technology Review, AI News, and arXiv.org provide up-to-date information on AI developments.
- Attend AI conferences or webinars to learn about the latest developments.
Many conferences now offer virtual attendance options, making them more accessible.
- Experiment with AI tools and platforms to gain hands-on experience.
Platforms like Google Colab or Azure ML Studio allow you to experiment with machine learning models without extensive programming knowledge.
- Engage in discussions about AI ethics and policy to understand the broader implications.
Join online forums or local meetups focused on AI ethics and governance.
- Consider taking online courses or pursuing certifications in AI-related fields.
Platforms like Coursera, edX, and Udacity offer a wide range of AI and machine learning courses.
Exercises to Deepen Your Understanding
To reinforce your learning about AI concepts, try these exercises:
- Choose an AI application you use regularly (e.g., a voice assistant or recommendation system) and research the AI techniques likely used in its development.
This will help you connect theoretical concepts to real-world applications.
- Identify a problem in your field or daily life that could potentially be solved with AI.
Sketch out a high-level plan for how you might approach this using AI techniques.
This exercise will help you think creatively about AI applications.
- Explore an open-source AI library like TensorFlow or PyTorch.
Try running a simple machine learning model on a sample dataset.
This hands-on experience will give you a better understanding of how AI models work in practice.
- Analyze a recent news article about AI.
Identify the key AI concepts mentioned and research any terms you’re unfamiliar with.
This will help you stay up-to-date with current AI developments and expand your AI vocabulary.
- Engage in a thought experiment: consider the potential implications if Artificial General Intelligence (AGI) were achieved. What potential benefits and risks do you foresee?
How might society need to adapt?
This exercise will help you think critically about the long-term implications of AI development.
Frequently Asked Questions
What is the difference between AI and machine learning?
Artificial Intelligence is a broad field that aims to create intelligent machines that can perform tasks requiring human-like intelligence. Machine learning is a subset of AI that focuses on developing algorithms that can learn from and make predictions or decisions based on data.
How is deep learning different from traditional machine learning?
Deep learning uses neural networks with many layers (hence “deep”) to automatically learn features from data. Traditional machine learning often needs manual feature engineering.
Deep learning can handle more complex patterns and has shown remarkable performance in tasks like image and speech recognition.
Can AI become conscious or self-aware?
This is a complex philosophical question without a clear scientific consensus. Current AI systems are not conscious or self-aware in the way humans are.
The possibility of machine consciousness stays a topic of debate among researchers and philosophers.
What are some ethical concerns surrounding AI development?
Key ethical concerns include AI bias and fairness, privacy issues, job displacement, and the potential for AI to be used in harmful ways (e.g., autonomous weapons). Ensuring responsible AI development that benefits humanity is a major focus of AI ethics research.
How is AI being used in healthcare?
AI is being used in healthcare for tasks such as medical image analysis, drug discovery, personalized treatment planning, and predictive analytics for patient outcomes. It’s helping to improve diagnosis accuracy and efficiency in many areas of medicine.
What is natural language processing (NLP) and how is it used?
NLP is a branch of AI that focuses on enabling computers to understand, interpret, and generate human language. It’s used in applications like chatbots, language translation, sentiment analysis, and voice assistants.
How might AI impact future job markets?
AI is expected to automate many tasks, potentially displacing some jobs. However, it’s also likely to create new job categories and enhance productivity in many fields.
The key will be adapting workforce skills to complement AI capabilities.
What is the role of big data in AI development?
Big data plays a crucial role in AI development by providing the large datasets necessary to train complex AI models. The more data available, the better AI systems can learn and improve their performance.
How secure are AI systems against hacking or manipulation?
AI security is an important and evolving field. Like any computer system, AI can be vulnerable to hacking or manipulation, especially if proper security measures aren’t in place.
Researchers are working on developing more robust and secure AI systems.
What is federated learning and why is it important?
Federated learning is a machine learning technique that trains algorithms across many decentralized devices or servers holding local data samples, without exchanging them. This approach helps preserve privacy and can be useful in scenarios where data cannot be centralized because of privacy concerns or regulations.
Key Takeaways
- AI encompasses a range of technologies including machine learning, neural networks, and natural language processing.
- Machine learning, especially deep learning, is driving many of the recent advancements in AI.
- AI is transforming various industries, from healthcare and finance to education and creative arts.
- Ethical considerations, including bias, privacy, and job displacement, are crucial as AI becomes more pervasive.
- Emerging frontiers like explainable AI, AGI, and quantum computing are shaping the future of AI research.
- Staying informed about AI developments is crucial for navigating our increasingly AI-driven world.
- Hands-on experience and critical thinking about AI’s implications can deepen understanding of this complex field.
A time-saving step to learning and applying the tools needed to utilize artificial intelligence in whatever application you are using it for is found at this link: https://galaxy.ai/?ref=gary
This post contains links. If you click on these links and make a purchase, I may earn a commission at no additional cost to you. Rest assured, I only recommend products or services I believe will add value to my readers.
Articles similar to this one can be found at: https://aiismsforbeginners.com/